Personalised and Adaptive Radiotherapy
Theme 1 is focusing on accurate detection of organ motion and patients’ outcome utilising advanced imaging and artificial intelligent in radiotherapy pathways.
Theme Leads
Dr Ann Henry (Co-Lead)
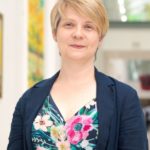
Associate Professor, Clinical Oncology
Staff Profile: Dr Ann Henry | School of Medicine | University of Leeds
Dr Bashar Al-Qaisieh (Co-Lead)
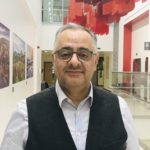
Staff Profile: Dr Bashar Al-Qaisieh | Lead Radiotherapy Imaging, R&D | Leeds Cancer Centre
Lead in Radiotherapy Imaging
Work Package 1 – Intelligent Treatment Motion Adaption
Work package 1 is designing a neural network architecture to predict real-time 4D motion from a sequence of 2D projection images, using graph convolutional networks. This will allow prediction and compensation for organ motion during radiotherapy delivery to optimise patient outcomes by reducing normal tissue irradiation and avoiding geographical miss.
Other WP1 Researchers
Dr Zeike Taylor
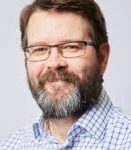
Associate Professor in Mechanical Engineering
Staff profile: Dr Zeike Taylor | School of Mechanical Engineering | University of Leeds
Dr Michael Nix
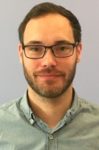
Medical Physicist
Dr Mike Nix is a RadNet Principal Clinical R&D Scientist and an honorary researcher at University of Leeds, where he is leading development of innovative deep-learning methodologies for radiotherapy imaging and adaptive therapy, alongside tools for clinical confidence estimation and effective translation.
Within Leeds Teaching Hospitals NHS Trust, he leads the development, evaluation and implementation of clinical AI technologies in Radiotherapy, in collaboration with commercial partners.
He is also a Clinical Fellow with Health Education England and NHSx, researching skills-gaps and developing educational strategy around robust and confident AI implementation for healthcare, following a year as one of the inaugural NHS Topol Fellows in Digital Health.
Dr Arezoo Zakeri
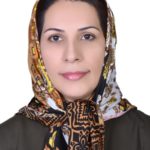
Post Doc Research Fellow
Staff profile: Dr Arezoo Zakeri | School of Computing | University of Leeds
Work Package 2 – Multifaceted Treatment Personalisation
Work package 2 has established proof-of-concept for use of distributed learning for predicting outcomes in rare cancers. This is being extended to a large consortium of centres examining outcomes after radiotherapy for anal cancer, including more complex data such as radiomics and patient reported outcomes. We are also developing deep learning methodology for predicting toxicity outcomes after pelvic radiotherapy.
Other WP2 Researchers
Dr Ane Appelt
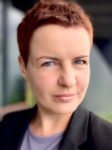
Associate Professor, Radiotherapy Physics
Staff Profile: Dr Ane Appelt | School of Medicine | University of Leeds
Dr Alex Gilbert
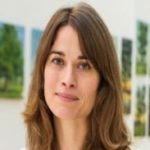
Honorary Consultant, Senior Clinical Trials Fellow
Staff Profile: Dr Alex Gilbert | School of Medicine | University of Leeds